Introduction
Artificial intelligence (AI) is reshaping the landscape of engineering and product design, ushering in a new era of innovation and efficiency. Over the past 15 years, AI has evolved from a promising concept to a powerful tool that is revolutionizing traditional design processes. This comprehensive review explores the transformative impact of AI on design, examining both traditional and modern AI methods. From expert systems, fuzzy logic, and genetic algorithms to machine learning and deep learning, AI techniques are enhancing every stage of the design process, from inspiration to modeling.
The growing interest in data-driven design methods and Explainable Artificial Intelligence (XAI) underscores the increasing relevance of AI in design applications. AI’s ability to manage complex design operations, optimize decision-making, and generate innovative solutions is setting new benchmarks in design efficiency and accuracy. However, choosing the right AI method tailored to specific design challenges is crucial for successful outcomes.
This blog post aims to provide designers, researchers, and practitioners with valuable insights into the diverse applications of AI in engineering and product design. Through this exploration, readers will gain a deeper understanding of the strengths, limitations, and future potential of AI-assisted design, setting the stage for a more innovative and collaborative design future.
Traditional vs. Modern AI Methods in Design
The journey of AI in design can be traced back to the utilization of traditional AI methods such as expert systems, fuzzy logic, and genetic algorithms. These rule-based techniques have long been the cornerstone of design evaluation and optimization, providing structured approaches to complex design challenges. Expert systems, for instance, emulate human expertise by using a knowledge base and a set of rules to solve specific problems. Fuzzy logic, on the other hand, allows for reasoning with imprecise or uncertain information, making it well-suited for design scenarios with ambiguous parameters. Genetic algorithms mimic the process of natural selection to optimize design solutions, evolving and adapting over multiple iterations to find the most efficient solutions.
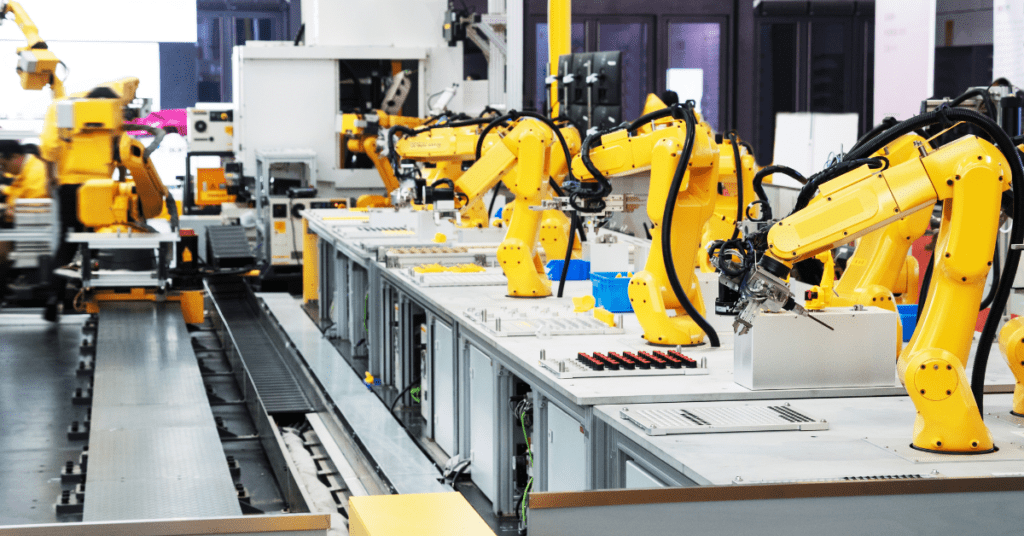
In contrast, the advent of modern data-driven AI techniques like machine learning and deep learning has brought about a paradigm shift in the design process. Machine learning algorithms can analyze large datasets to identify patterns and make predictions, enabling designers to derive insights and make informed decisions. Deep learning, a subset of machine learning, uses neural networks with multiple layers to process complex data and extract meaningful information, offering unprecedented capabilities in image recognition, natural language processing, and predictive modeling.
Over the past 15 years, the integration of these modern AI methods into the design process has accelerated, enabling designers to tackle complex design problems with greater precision and creativity. These data-driven AI techniques not only complement traditional rule-based methods but also extend the boundaries of what is possible in design, paving the way for innovative solutions and transformative design experiences. As AI continues to evolve and mature, the synergy between traditional and modern AI methods promises to unlock new opportunities and redefine the future of engineering and product design.
AI-Powered Design Across Various Stages
AI’s transformative impact on the design process extends across multiple stages, reshaping the way designers’ approach and solve complex problems.
Inspiration and Idea Generation: AI algorithms can analyze vast amounts of design data, trends, and patterns to inspire and generate innovative design concepts. By identifying hidden connections and relationships within the data, AI can offer designers fresh perspectives and novel ideas that might not be apparent through traditional methods alone.
Evaluation and Optimization: Traditional AI methods like genetic algorithms and fuzzy logic excel in evaluating and optimizing design solutions. Genetic algorithms simulate natural evolution to find optimal design solutions by iteratively selecting, combining, and mutating design parameters. Fuzzy logic enables designers to make decisions based on imprecise or uncertain information, allowing for more flexible and adaptive design evaluations.
The advent of machine learning and deep learning has further enhanced the evaluation and optimization stages. Machine learning algorithms can analyze and learn from past design data to predict and optimize future design outcomes. Deep learning techniques, with their ability to process and interpret complex data structures, offer unprecedented capabilities in design optimization by identifying subtle patterns and correlations that human designers might overlook.
Decision-Making and Modeling: AI-powered decision-making tools assist designers in making informed decisions by providing data-driven insights and recommendations. These tools can evaluate multiple design alternatives, assess their feasibility, and predict their performance, enabling designers to make more informed and confident decisions.
In the modeling stage, AI techniques like artificial neural networks and deep learning algorithms can generate accurate and detailed design models based on input parameters and constraints. These AI-driven modeling tools can significantly streamline the design process, reduce manual labor, and improve the accuracy and consistency of design models.
The Future of AI in Design: As AI continues to advance, the integration of AI across various design stages is expected to become more seamless and sophisticated. The combination of AI with emerging technologies like augmented reality, virtual reality, and 3D printing holds the potential to revolutionize the design process further. AI-driven design tools and platforms will become increasingly collaborative, intuitive, and user-friendly, empowering designers to explore new design possibilities, enhance creativity, and deliver innovative solutions that meet the evolving needs and expectations of users and stakeholders.
In conclusion, AI’s growing influence across the design process is reshaping the future of engineering and product design, offering unprecedented opportunities to enhance efficiency, creativity, and innovation. By embracing AI-powered design tools and methodologies, designers can unlock new possibilities, overcome complex challenges, and create designs that resonate with users, drive business success, and shape a more sustainable and inclusive future.
Growing Interest in Data-Driven Design and XAI
The increasing interest in data-driven design methods and Explainable Artificial Intelligence (XAI) highlights the evolving landscape of AI in design applications.
Data-Driven Design Methods: Data-driven design leverages the power of AI to analyze and interpret vast amounts of design data, enabling designers to derive actionable insights, identify patterns, and make informed decisions. By harnessing the potential of machine learning and deep learning algorithms, designers can explore new design possibilities, optimize design parameters, and predict design outcomes with unprecedented accuracy and efficiency. Data-driven design methods not only streamline the design process but also foster innovation by empowering designers to explore and experiment with new ideas and concepts.
Explainable Artificial Intelligence (XAI): As AI continues to evolve and integrate into various aspects of design, the need for transparency, interpretability, and trust in AI-driven design solutions becomes increasingly important. Explainable Artificial Intelligence (XAI) aims to make AI algorithms and decisions more understandable and interpretable to designers and stakeholders. By providing insights into the decision-making process of AI algorithms, XAI enables designers to gain a deeper understanding of AI-generated design solutions, evaluate their reliability and validity, and make more informed and confident design decisions.
The growing interest in XAI underscores the importance of building trust and fostering collaboration between AI and human designers. XAI not only enhances the transparency and interpretability of AI-driven design solutions but also promotes a more collaborative and inclusive design process by enabling designers and stakeholders to engage with AI algorithms, provide feedback, and co-create design solutions that align with their goals, preferences, and values.
In conclusion, the growing interest in data-driven design methods and Explainable Artificial Intelligence (XAI) is reshaping the way designers approach and engage with AI in the design process. By embracing data-driven design methodologies and integrating XAI into AI-driven design solutions, designers can enhance transparency, foster collaboration, and unlock new opportunities to innovate, create, and deliver design solutions that meet the evolving needs and expectations of users and stakeholders in today’s rapidly changing and increasingly complex design landscape.
Choosing the Right AI Method for Design Problems
Selecting the appropriate AI method tailored to specific design challenges is crucial for achieving successful outcomes in engineering and product design. With a plethora of AI techniques available, ranging from traditional rule-based methods to modern data-driven approaches, designers must carefully evaluate and choose the right AI method that aligns with their design objectives, constraints, and requirements.
Considerations for Choosing AI Methods:
- Nature of Design Problem: Different AI methods are suited to different types of design problems. For instance, rule-based methods like expert systems and fuzzy logic may be more appropriate for well-defined, structured design problems with clear rules and constraints, while data-driven methods like machine learning and deep learning may be better suited for complex, unstructured design problems with large datasets and patterns that are not easily discernible.
- Design Stage and Complexity: The choice of AI method can vary depending on the design stage and complexity of the problem. For example, genetic algorithms and optimization algorithms may be employed during the evaluation and optimization stages to refine and optimize design solutions, whereas machine learning and deep learning techniques may be utilized during the ideation and concept generation stages to explore and generate innovative design concepts.
- Available Data and Resources: The availability of data and computational resources can influence the choice of AI method. Data-driven methods require large datasets for training and validation, while rule-based methods may rely on expert knowledge and domain-specific rules. Additionally, the computational complexity and scalability of AI methods should be considered to ensure that the chosen method can efficiently handle the design problem and meet the required performance criteria.
- Interpretability and Transparency: The level of interpretability and transparency required for the design problem can also guide the selection of AI methods. For design problems where transparency and interpretability are crucial, Explainable Artificial Intelligence (XAI) methods should be considered to enhance the transparency and trustworthiness of AI-driven design solutions.
In conclusion, choosing the right AI method for design problems is a critical step in leveraging the power of AI to enhance the design process, optimize design solutions, and drive innovation. By carefully evaluating and selecting the appropriate AI method based on the nature of the design problem, design stage, available data and resources, and desired level of interpretability and transparency, designers can harness the full potential of AI to tackle complex design challenges, explore new design possibilities, and deliver innovative and impactful design solutions that meet the evolving needs and expectations of users and stakeholders.
Strengths and Weaknesses of AI Methods in Design
While AI methods offer transformative capabilities in engineering and product design, it is essential to understand their strengths and weaknesses to effectively leverage their potential and address their limitations.
Strengths of AI Methods:
- Efficiency and Speed: AI algorithms can analyze and process vast amounts of design data at incredible speeds, enabling designers to explore and evaluate numerous design alternatives quickly and efficiently.
- Accuracy and Consistency: AI-driven design solutions can provide accurate and consistent results by minimizing human error and variability, enhancing the reliability and repeatability of design outcomes.
- Innovation and Creativity: AI algorithms can generate novel and innovative design concepts by identifying hidden patterns and relationships within design data, fostering creativity and inspiring new design ideas and solutions.
- Optimization and Decision Support: AI methods like genetic algorithms and machine learning can optimize design parameters and support decision-making by evaluating multiple design alternatives, predicting their performance, and identifying the most optimal and feasible solutions.
Weaknesses of AI Methods:
- Lack of Domain Knowledge: AI algorithms may lack domain-specific knowledge and expertise, making them reliant on the quality and relevance of the input data and potentially limiting their ability to understand and address complex design challenges and constraints.
- Interpretability and Transparency: Some AI methods, especially deep learning algorithms, can be complex and difficult to interpret and explain, hindering designers’ understanding of the underlying decision-making process and reducing trust and confidence in AI-driven design solutions.
- Over-reliance on Data: Data-driven AI methods require large and high-quality datasets for training and validation, and their performance and reliability can be affected by the availability and quality of data, potentially leading to biased or inaccurate design outcomes.
- Computational Complexity and Scalability: Some AI methods, particularly optimization algorithms and deep learning techniques, can be computationally intensive and may require substantial computational resources and time to solve complex design problems, limiting their applicability and scalability in real-world design scenarios.
In conclusion, while AI methods offer significant advantages in enhancing efficiency, accuracy, innovation, and decision support in engineering and product design, it is crucial to recognize and address their limitations and challenges. By understanding the strengths and weaknesses of different AI methods and integrating them appropriately into the design process, designers can harness the full potential of AI to overcome complex design challenges, explore new design possibilities, and deliver innovative and impactful design solutions that meet the evolving needs and expectations of users and stakeholders.
Future Trends and Research Directions in AI-Assisted Design
As AI continues to advance and evolve, its integration into engineering and product design is expected to drive new trends, methodologies, and research directions that will further reshape the design landscape.
Integration of Emerging Technologies: The convergence of AI with emerging technologies such as augmented reality (AR), virtual reality (VR), and 3D printing holds immense potential to revolutionize the design process. AI-driven AR and VR tools can create immersive design environments, enabling designers to visualize and interact with design concepts in real-time, explore design alternatives, and evaluate design performance in virtual settings. Additionally, AI-powered 3D printing technologies can automate and optimize the fabrication process, enabling the rapid prototyping and production of complex and customized design solutions.
Advancements in Explainable AI (XAI): The growing importance of transparency, interpretability, and trust in AI-driven design solutions is driving research and advancements in Explainable Artificial Intelligence (XAI). Future developments in XAI are expected to enhance the interpretability and explainability of AI algorithms, enabling designers to gain deeper insights into AI-generated design solutions, evaluate their reliability and validity, and make more informed and confident design decisions.
Collaborative and Generative Design: AI-powered collaborative and generative design tools and platforms will become increasingly prevalent, fostering collaboration and co-creation between AI and human designers. These AI-driven tools can assist designers in exploring and generating innovative design concepts, optimizing design parameters, and providing real-time feedback and recommendations, enabling designers to explore new design possibilities, enhance creativity, and deliver design solutions that resonate with users and stakeholders.
Ethical and Responsible AI Design: As AI continues to play a significant role in shaping the design landscape, there is an increasing emphasis on ethical and responsible AI design practices. Future research and developments will focus on addressing ethical considerations, ensuring fairness, transparency, and accountability in AI-driven design solutions, and promoting responsible AI design practices that prioritize the well-being, inclusivity, and sustainability of design solutions and their impact on society and the environment.
In conclusion, the future of AI-assisted design is poised to be characterized by the integration of emerging technologies, advancements in Explainable AI (XAI), collaborative and generative design methodologies, and a focus on ethical and responsible AI design practices. By embracing these trends and research directions, designers can harness the full potential of AI to innovate, create, and deliver design solutions that meet the evolving needs and expectations of users and stakeholders in today’s rapidly changing and increasingly complex design landscape.
Conclusion
The transformative impact of artificial intelligence (AI) on engineering and product design over the past 15 years has been nothing short of revolutionary. From traditional rule-based methods to modern data-driven techniques, AI has reshaped the design landscape, offering designers unprecedented capabilities to tackle complex challenges, optimize design solutions, and drive innovation.
By leveraging the strengths of AI in efficiency, accuracy, creativity, and decision support, designers can streamline the design process, explore new design possibilities, and deliver impactful solutions that resonate with users and stakeholders. However, it is equally important to recognize and address the limitations and challenges of AI methods, such as the lack of domain knowledge, interpretability issues, data reliance, and computational complexity, to effectively harness the full potential of AI in real-world design scenarios.
Looking ahead, the future of AI-assisted design holds exciting opportunities and challenges, characterized by the integration of emerging technologies like augmented reality, virtual reality, and 3D printing, advancements in Explainable AI (XAI), collaborative and generative design methodologies, and a focus on ethical and responsible AI design practices. By embracing these trends, methodologies, and research directions, designers can unlock new possibilities, overcome complex challenges, and create innovative and sustainable design solutions that meet the evolving needs and expectations of users and stakeholders in today’s rapidly changing and increasingly complex design landscape.
In conclusion, while AI continues to evolve and reshape the design landscape, the human-centric approach to design remains paramount. By fostering collaboration between AI and human designers, embracing ethical and responsible AI design practices, and prioritizing transparency, interpretability, and trust in AI-driven design solutions, designers can harness the full potential of AI to innovate, create, and deliver design solutions that enhance the quality of life, drive business success, and shape a more sustainable, inclusive, and resilient future for all.
Related Content
- Review of artificial intelligence applications in engineering design perspective
- A grouping method for optimization of steel skeletal structures by applying a combinatorial search algorithm based on a fully stressed design
- Three-dimensional free bending vibrations of variable radius functionally graded circular column immersed in infinite fluid
Explore Micro2media.com
- Rolling the Dice: Navigating Probability Judgement in Artificial Intelligence
- Revolutionizing Tomorrow: The Rise of ChatGPT and the Future of AI-Powered Conversations
- Navigating the Future: Ethical Considerations in AI and Robotics
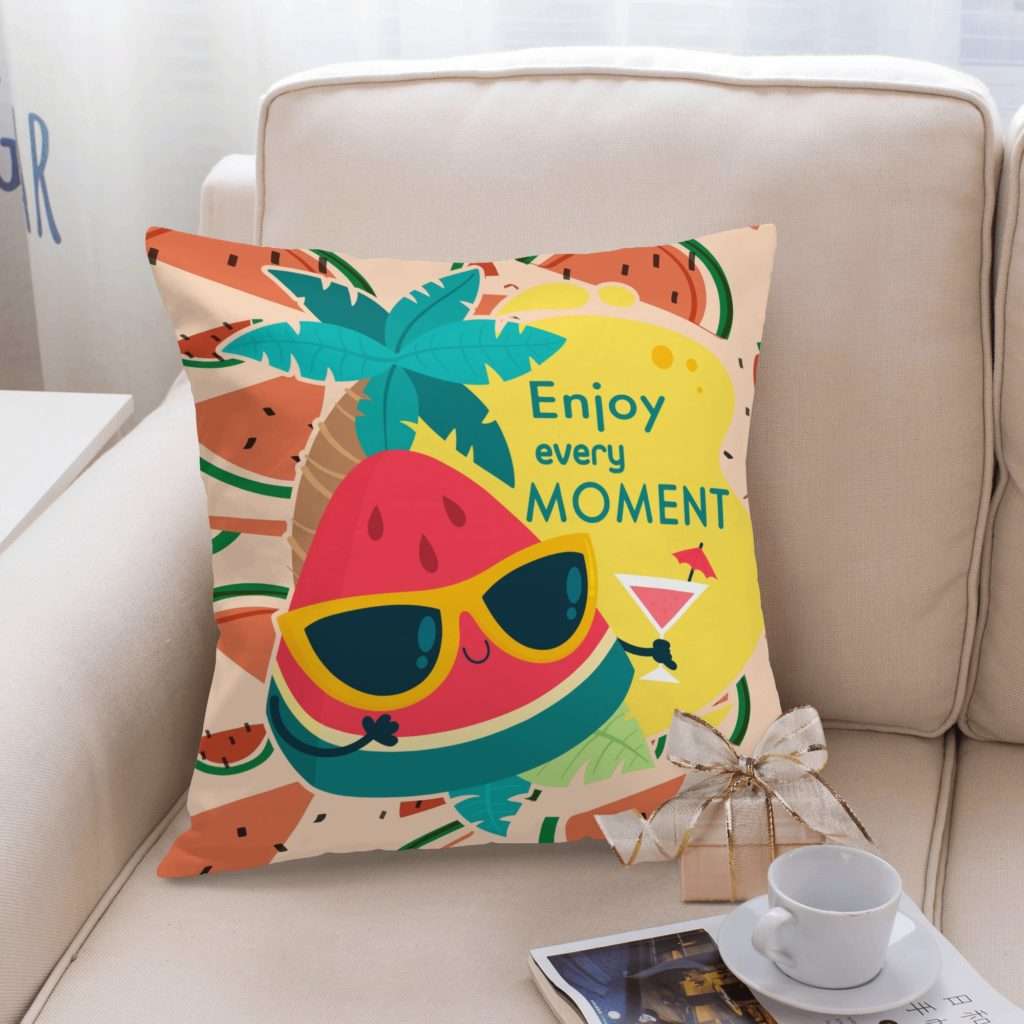