Introduction
Artificial Intelligence (AI) is rapidly transforming various sectors, and healthcare is no exception. Among the many applications of AI in medicine, predictive analytics stands out as a game-changer. By analyzing vast amounts of data, predictive analytics can forecast health trends, identify potential risks, and suggest preventive measures. This technology holds the promise of revolutionizing preventive care, making it possible to detect diseases early, optimize treatment plans, and improve patient outcomes. In this article, we will explore how AI-predictive analytics is ushering in a new era of prevention in healthcare, its applications, benefits, challenges, and future trends.
Understanding AI-Predictive Analytics
Definition and Basic Principles
AI-predictive analytics refers to the use of artificial intelligence technologies to analyze data and make predictions about future events or trends. In healthcare, this involves using complex algorithms and machine learning models to sift through patient data, identify patterns, and predict potential health outcomes. This predictive power enables healthcare providers to take proactive measures, potentially saving lives and reducing healthcare costs.
Historical Development and Advancements
The journey of predictive analytics in healthcare began with simple statistical models. Over time, advancements in AI and machine learning have significantly enhanced the capabilities of these models. Today, predictive analytics leverages sophisticated algorithms that can process large datasets in real-time, offering insights that were previously unimaginable. This evolution has paved the way for more accurate and actionable predictions.
Types of Predictive Analytics
- Descriptive Analytics: This type involves analyzing historical data to understand past trends and patterns. It sets the foundation for more advanced predictive models.
- Predictive Analytics: This is the core focus, using statistical models and machine learning techniques to predict future outcomes based on historical data.
- Prescriptive Analytics: This advanced form not only predicts outcomes but also suggests actions to achieve desired results, thereby guiding decision-making processes in healthcare settings.
Applications of Predictive Analytics in Healthcare
Early Disease Detection
One of the most significant applications of predictive analytics is early disease detection. By analyzing patient records, genetic information, and lifestyle data, predictive models can identify individuals at high risk for diseases such as cancer, diabetes, and cardiovascular conditions. This early detection allows for timely intervention, improving the chances of successful treatment and reducing the burden on healthcare systems. For more details, read this article on early disease detection using AI.
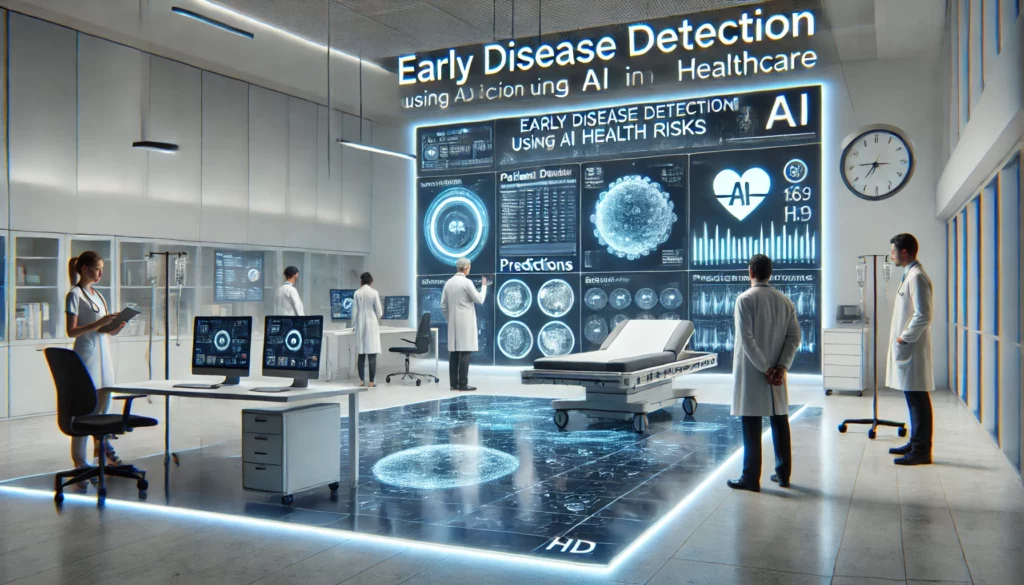
Predictive Modeling for Patient Outcomes
Predictive analytics can forecast patient outcomes, helping healthcare providers tailor treatments to individual needs. For instance, predictive models can estimate the likelihood of complications following surgery, enabling doctors to take preventive measures. Similarly, they can predict the progression of chronic diseases, allowing for better management and improved patient quality of life.
Resource Management and Optimization
Healthcare facilities often face challenges related to resource allocation and management. Predictive analytics can optimize these processes by forecasting patient admissions, staffing needs, and inventory requirements. This ensures that resources are used efficiently, reducing waste and improving overall service delivery.
Personalized Treatment Plans
AI-predictive analytics can contribute to the development of personalized treatment plans. By analyzing a patient’s unique genetic makeup, medical history, and lifestyle factors, predictive models can recommend the most effective treatment options. This personalized approach enhances patient care and increases the likelihood of positive outcomes.
Benefits of Predictive Analytics in Prevention
Reducing Hospital Readmissions
Predictive analytics plays a crucial role in reducing hospital readmissions by identifying patients at high risk of complications or relapse. By analyzing patient data, healthcare providers can implement targeted interventions, such as follow-up appointments, medication adjustments, and lifestyle recommendations. This proactive approach not only improves patient outcomes but also reduces the financial burden on healthcare systems.
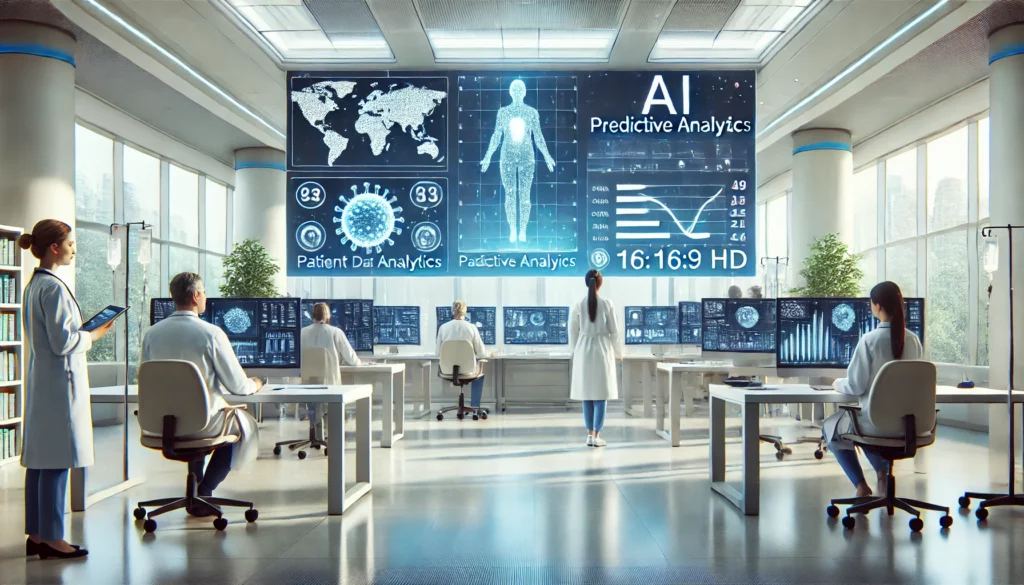
Improving Patient Outcomes
The ability to predict health issues before they become critical allows for timely and effective interventions. Predictive analytics enables healthcare providers to anticipate potential health problems and take preventive measures, leading to improved patient outcomes. Early detection of diseases, personalized treatment plans, and continuous monitoring all contribute to better health and quality of life for patients.
Cost-Effectiveness and Resource Allocation
Predictive analytics helps optimize the allocation of healthcare resources, ensuring they are used where they are most needed. By predicting patient influx, resource utilization, and potential bottlenecks, healthcare facilities can streamline operations and reduce costs. This efficient resource management leads to better service delivery and financial sustainability.
Enhancing Patient Engagement and Satisfaction
Predictive analytics fosters a more personalized approach to patient care, enhancing patient engagement and satisfaction. By tailoring treatment plans and preventive measures to individual needs, healthcare providers can offer more effective and patient-centered care. This personalized attention builds trust and encourages patients to take an active role in managing their health.
Case Studies and Real-World Examples
Successful Implementations in Various Healthcare Settings
Several healthcare institutions have successfully implemented predictive analytics to improve patient care and operational efficiency. For example, hospitals using predictive models for early sepsis detection have seen a significant reduction in mortality rates. Similarly, predictive analytics has been used to manage chronic diseases like diabetes, resulting in better patient outcomes and reduced healthcare costs. For a detailed case study, visit this link.
Analysis of Data from Pilot Programs and Studies
Numerous pilot programs and studies have demonstrated the effectiveness of predictive analytics in healthcare. For instance, a study on predictive analytics for heart disease prevention showed a notable decrease in the incidence of heart attacks among high-risk patients. These studies provide valuable insights and highlight the potential of predictive analytics to transform healthcare delivery.
Testimonials from Healthcare Professionals
Healthcare professionals who have adopted predictive analytics often report positive experiences. Many emphasize the improved accuracy of diagnoses, better patient management, and enhanced decision-making capabilities. These testimonials underscore the practical benefits of predictive analytics and encourage wider adoption in the healthcare industry.
Challenges and Ethical Considerations
Data Privacy and Security Concerns
One of the major challenges in implementing predictive analytics in healthcare is ensuring data privacy and security. Patient data is highly sensitive, and any breach can have severe consequences. Healthcare providers must adhere to strict data protection regulations and employ robust security measures to safeguard patient information.
Ethical Dilemmas in AI Decision-Making
The use of AI in healthcare raises several ethical dilemmas, particularly concerning decision-making. There are concerns about the transparency of AI algorithms, potential biases in predictive models, and the accountability for AI-driven decisions. Addressing these ethical issues is crucial to build trust and ensure the responsible use of AI in healthcare. Learn more about ethical issues in AI here.
Potential Biases in Predictive Models
Predictive models can sometimes exhibit biases, leading to inaccurate predictions and unfair treatment of certain patient groups. These biases can stem from various sources, including biased training data and flawed algorithm design. Continuous monitoring and refinement of predictive models are essential to minimize biases and ensure fair and equitable healthcare delivery. For more information on addressing biases in AI, refer to this study.
Regulatory and Compliance Issues
The use of predictive analytics in healthcare is subject to various regulatory and compliance requirements. Navigating these regulations can be challenging, and healthcare providers must stay updated on the latest legal standards. Ensuring compliance is essential to avoid legal repercussions and maintain the integrity of predictive analytics initiatives.
Future Trends in AI-Predictive Analytics
Emerging Technologies and Innovations
The future of AI-predictive analytics in healthcare is bright, with numerous emerging technologies poised to enhance its capabilities. Innovations in machine learning, natural language processing, and big data analytics will drive the development of more sophisticated predictive models, offering even greater accuracy and actionable insights.
Predictions for the Next Decade
Over the next decade, we can expect predictive analytics to become even more integrated into healthcare systems. Real-time data analysis, predictive maintenance of medical equipment, and AI-driven patient care will likely become standard practices. These advancements will further improve patient outcomes and healthcare efficiency.
The Role of Government and Policy in Shaping the Future
Government policies and regulations will play a crucial role in shaping the future of AI-predictive analytics in healthcare. Ensuring that ethical standards are maintained and that patient data is protected will be paramount. Collaborative efforts between policymakers, healthcare providers, and technology developers will be essential to harness the full potential of predictive analytics.
Conclusion
The integration of AI-predictive analytics in healthcare marks a significant advancement in medical science, ushering in a new era of prevention and personalized care. By leveraging the power of AI, healthcare providers can predict potential health issues before they become critical, enabling early interventions and improving patient outcomes. The benefits of predictive analytics are far-reaching, from reducing hospital readmissions and optimizing resource allocation to enhancing patient engagement and satisfaction.
However, the journey to fully realize the potential of predictive analytics is not without its challenges. Ensuring data privacy and security, addressing ethical dilemmas, and mitigating biases in predictive models are critical issues that must be tackled. Additionally, navigating the complex landscape of regulatory and compliance requirements is essential to maintain the integrity of predictive analytics initiatives.
As we look to the future, the role of AI in healthcare is set to expand further. Emerging technologies and innovations will continue to drive advancements in predictive analytics, offering even more precise and actionable insights. The collaborative efforts of healthcare professionals, policymakers, and technology developers will be crucial in shaping a future where predictive analytics becomes an integral part of healthcare delivery.
In conclusion, AI-predictive analytics holds the promise of transforming healthcare by enabling a proactive approach to prevention and personalized care. By embracing this technology, we can move towards a healthier future where early detection and tailored treatments become the norm, ultimately improving the quality of life for patients worldwide.
FAQs
1. How does predictive analytics improve early disease detection?
Predictive analytics uses AI algorithms to analyze large datasets, including patient records and genetic information, to identify patterns and risk factors for diseases. This early identification allows for timely interventions, potentially preventing diseases from progressing. For more details, read this article on early disease detection using AI.
2. What are some successful case studies of predictive analytics in healthcare?
Several hospitals and healthcare institutions have successfully implemented predictive analytics. For example, predictive models for early sepsis detection have significantly reduced mortality rates. Another case study demonstrated a decrease in heart attacks among high-risk patients using predictive analytics. For a detailed case study, visit this link.
3. What ethical considerations are associated with AI in healthcare?
The use of AI in healthcare raises ethical concerns such as data privacy, transparency in AI decision-making, and potential biases in predictive models. Ensuring that AI algorithms are transparent and free from biases is essential to maintain trust and fairness in healthcare. Learn more about ethical issues in AI here.
4. How can healthcare providers address potential biases in predictive models?
Healthcare providers can address biases in predictive models by continuously monitoring and refining the algorithms. Using diverse and representative datasets during the training phase can also help mitigate biases. For more information on addressing biases in AI, refer to this study.