Introduction
In an era where data is hailed as the new oil, predictive analytics stands out as a crucial tool for extracting actionable insights from vast datasets. Predictive analytics involves using statistical algorithms and machine learning techniques to identify the likelihood of future outcomes based on historical data. This practice has been a game-changer across various industries, enabling businesses to anticipate trends, optimize operations, and make informed decisions. However, the advent of artificial intelligence (AI) has significantly elevated the capabilities of predictive analytics. AI introduces a new dimension of accuracy, speed, and efficiency, allowing for more sophisticated and real-time predictions. From finance to healthcare, AI-powered predictive analytics is reshaping how organizations interpret data and strategize for the future. This article delves into how AI is transforming predictive analytics, exploring its benefits, challenges, and the promising future it holds.
Understanding Predictive Analytics
Predictive analytics is the process of using historical data to predict future events. It involves techniques such as data mining, statistical modeling, and machine learning to analyze current and historical facts to make predictions about future or otherwise unknown events. The key components of predictive analytics include data collection, data analysis, and predictive modeling. Before the integration of AI, predictive analytics relied heavily on traditional statistical methods and simpler machine learning algorithms. These methods, while effective to an extent, often required extensive manual data preprocessing and were limited in handling large datasets or complex patterns. Predictive analytics has found applications across various sectors: In finance, predictive models are used for credit scoring, fraud detection, and risk management. In healthcare, predictive analytics helps in predicting disease outbreaks, patient readmissions, and personalized treatment plans. In marketing, businesses use predictive analytics to forecast sales, understand customer behavior, and optimize marketing campaigns.
The Role of AI in Predictive Analytics
Artificial Intelligence, particularly machine learning and deep learning, has revolutionized predictive analytics. Machine learning algorithms learn from data and improve their predictions over time without being explicitly programmed. Deep learning, a subset of machine learning, uses neural networks with many layers to analyze complex patterns in large datasets. AI significantly enhances predictive analytics in several ways. Firstly, AI algorithms can uncover intricate patterns and relationships in data, leading to more accurate predictions. Secondly, AI systems can process and analyze large datasets much faster than traditional methods. Thirdly, the automation of data preprocessing and model training reduces the need for manual intervention, making the process more efficient. Case studies showcasing AI-driven predictive analytics include JPMorgan Chase using AI-powered predictive models to detect fraudulent transactions with higher accuracy, IBM Watson Health employing AI to predict patient outcomes and suggest personalized treatment options, and Netflix using AI-driven predictive analytics to recommend personalized content to its users, enhancing user engagement and retention.
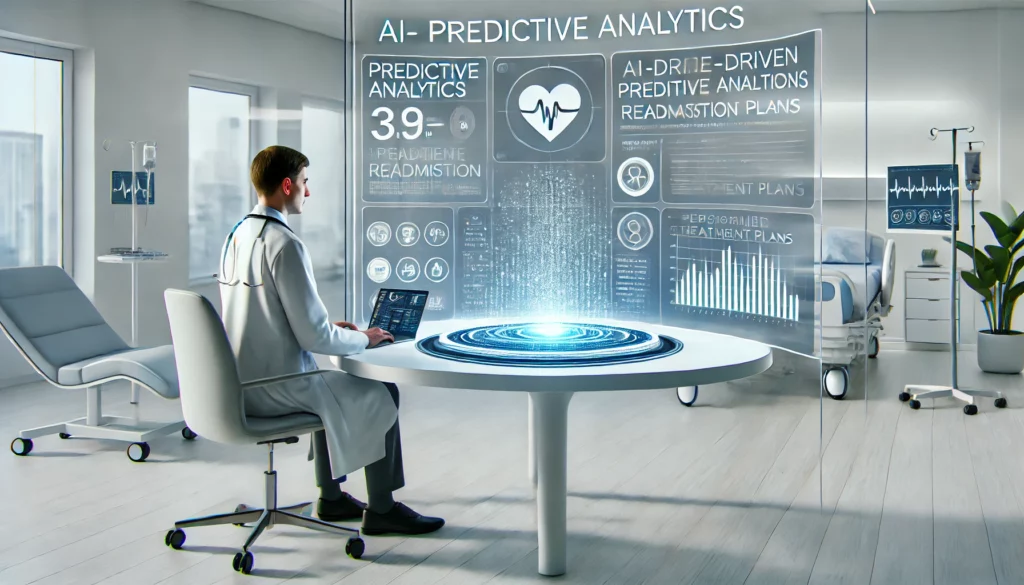
Key Benefits of AI in Predictive Analytics
The integration of AI into predictive analytics brings several key benefits. Improved data processing and analysis are among the foremost advantages. AI can handle vast amounts of data quickly and accurately, identifying patterns and trends that would be impossible for humans to detect. This leads to enhanced predictive models and insights, providing businesses with more reliable information for decision-making. Real-time predictions and decision-making are another critical benefit. AI systems can analyze data as it is generated, offering up-to-the-minute insights that enable organizations to respond swiftly to emerging trends and potential issues. This is particularly valuable in industries like finance and healthcare, where timely information can significantly impact outcomes. Additionally, AI-driven predictive analytics can lead to cost reduction and resource optimization. By automating complex data analysis tasks, organizations can save time and resources, reducing the need for extensive human intervention and allowing staff to focus on more strategic activities.
Challenges and Considerations
Despite its many advantages, the integration of AI into predictive analytics is not without challenges. Data privacy and security concerns are paramount, as the use of AI involves processing large amounts of sensitive information. Ensuring that this data is protected against breaches and misuse is critical. Ethical implications of AI in predictive analytics also need to be considered. The potential for bias in AI algorithms, the transparency of AI decision-making processes, and the broader societal impact of AI-driven decisions are all important factors that organizations must address. Technological and operational challenges can also pose significant hurdles. Implementing AI systems requires substantial investment in technology and expertise, and maintaining these systems can be complex and resource-intensive. Strategies to address these challenges include robust data governance frameworks, ongoing monitoring and evaluation of AI systems, and fostering a culture of transparency and ethical awareness within organizations.
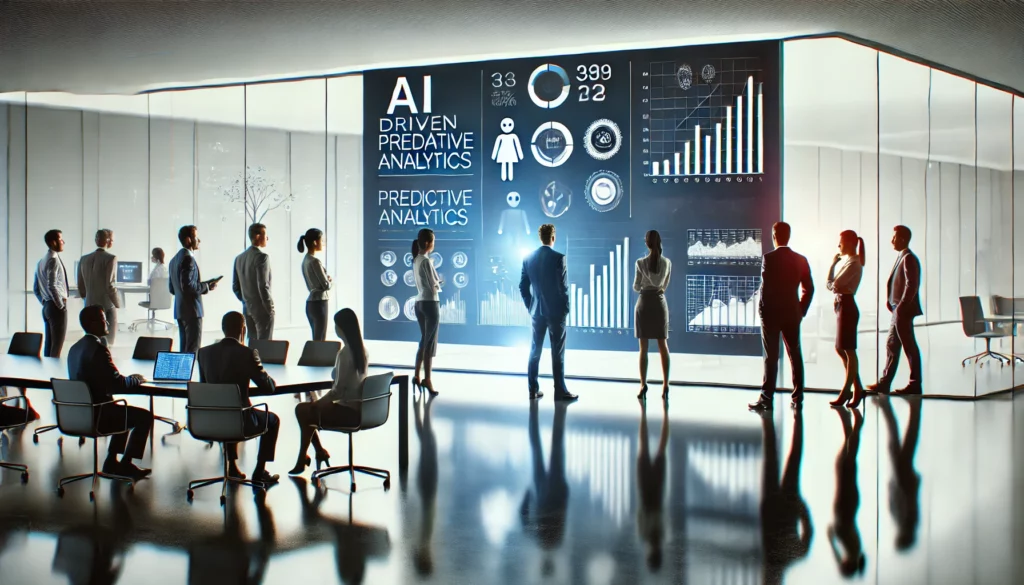
Future Trends and Developments
The future of predictive analytics with AI is filled with exciting possibilities. Emerging AI technologies, such as advanced natural language processing and enhanced neural networks, are set to further revolutionize predictive analytics. These technologies will enable even more sophisticated data analysis and more accurate predictions. The potential future landscape of AI in predictive analytics is one where real-time, highly accurate insights become the norm across industries. Businesses will increasingly rely on AI-driven predictive analytics to stay competitive, optimize operations, and deliver personalized experiences to customers. Predictions on the evolution of predictive analytics with AI suggest that we will see more integrated and seamless AI solutions, with predictive analytics becoming an integral part of everyday business operations. As AI technology continues to advance, its impact on predictive analytics will only grow, driving innovation and transformation across sectors.
Conclusion
In summary, the integration of AI into predictive analytics represents a significant advancement in how organizations leverage data to make informed decisions. AI enhances predictive analytics by improving accuracy, speed, and efficiency, enabling real-time insights and more sophisticated data analysis. While challenges such as data privacy, ethical considerations, and technological hurdles exist, the benefits of AI-driven predictive analytics are undeniable. As AI technologies continue to evolve, the future of predictive analytics looks promising, with endless possibilities for innovation and transformation. By harnessing the power of AI, businesses can unlock the full potential of predictive analytics, driving better outcomes and staying ahead in an increasingly data-driven world.
FAQs
1. What is predictive analytics and how is AI enhancing it?
Predictive analytics involves using historical data and statistical algorithms to forecast future events. AI enhances predictive analytics by improving the accuracy, speed, and efficiency of these forecasts. AI algorithms can analyze larger datasets and uncover complex patterns, leading to more precise predictions and real-time decision-making.
2. What are some real-world applications of AI-driven predictive analytics?
AI-driven predictive analytics is used in various sectors. In finance, it helps in fraud detection and credit scoring. In healthcare, it predicts patient outcomes and personalizes treatment plans. In marketing, it forecasts sales and understands customer behavior. Companies like JPMorgan Chase, IBM Watson Health, and Netflix are leading examples of AI-driven predictive analytics applications.
3. What challenges are associated with integrating AI into predictive analytics?
The integration of AI into predictive analytics presents several challenges, including data privacy and security concerns, ethical implications, and technological and operational hurdles. Ensuring data protection, addressing bias in AI algorithms, and managing the complexity of AI systems are critical issues that organizations must navigate.
4. What future trends can we expect in AI-driven predictive analytics?
Future trends in AI-driven predictive analytics include the development of advanced natural language processing and enhanced neural networks. These technologies will enable more sophisticated data analysis and highly accurate predictions. The future landscape will likely see real-time insights becoming standard, with predictive analytics playing a central role in business operations.