Artificial intelligence (AI) is transforming the world of finance in unprecedented ways. From banking to insurance, from wealth management to trading, AI is enabling financial institutions and investors to make smarter, faster, and more efficient decisions. AI is also creating new opportunities and challenges for the financial sector, as it reshapes the competitive landscape, the regulatory environment, and the ethical standards.
One of the key areas where AI is making a significant impact is in predictive analytics and algorithmic trading. Predictive analytics is the process of using data, statistical models, and machine learning techniques to forecast future outcomes and trends. Algorithmic trading is the use of computer programs and algorithms to execute trading orders based on predefined rules and strategies.
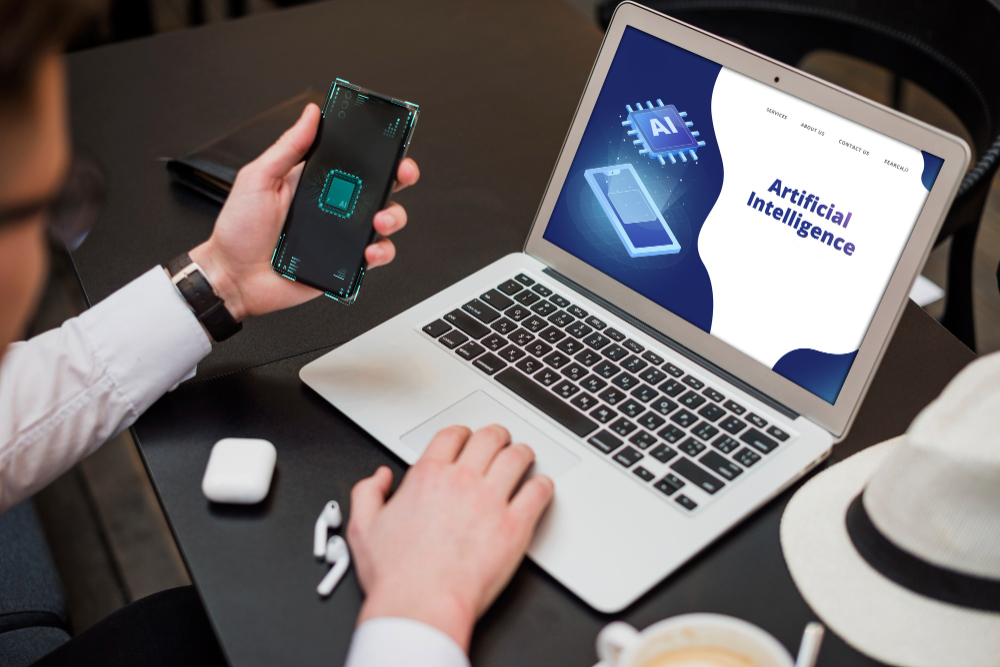
In this blog post, we will explore how AI-powered predictive analytics and algorithmic trading strategies are reshaping the financial landscape. We will examine the evolution of AI in finance, the applications and benefits of predictive analytics and algorithmic trading, the leading AI-driven platforms and tools, the regulatory and ethical considerations, and the future trends and outlook.
The Evolution of AI in Finance
AI is not a new phenomenon in finance. In fact, the history of AI adoption in finance can be traced back to the 1950s, when the first computerized trading systems were developed. Since then, AI has evolved and advanced significantly, thanks to the improvements in computing power, data availability, and algorithmic sophistication.
Some of the key developments and advancements in AI technologies that have influenced the finance industry are:
- Expert systems: These are computer programs that emulate the knowledge and reasoning of human experts in a specific domain. Expert systems were widely used in finance in the 1980s and 1990s, for tasks such as credit scoring, fraud detection, and portfolio management.
- Neural networks: These are computational models that mimic the structure and function of biological neurons. Neural networks are capable of learning from data and performing complex nonlinear operations. Neural networks have been applied in finance since the late 1980s, for tasks such as stock market prediction, option pricing, and risk analysis.
- Natural language processing (NLP): This is the branch of AI that deals with the analysis and generation of natural language. NLP enables computers to understand, interpret, and communicate with human language. NLP has been used in finance since the early 2000s, for tasks such as sentiment analysis, news mining, and chatbots.
- Machine learning (ML): This is the subfield of AI that focuses on creating systems that can learn from data and improve their performance without explicit programming. Machine learning encompasses a variety of techniques, such as supervised learning, unsupervised learning, reinforcement learning, and deep learning. Machine learning has been widely adopted in finance since the mid-2000s, for tasks such as anomaly detection, customer segmentation, and algorithmic trading.
- Deep learning (DL): This is a subset of machine learning that uses multiple layers of neural networks to learn from large amounts of data and perform complex tasks. Deep learning has emerged as a powerful tool in finance since the early 2010s, for tasks such as image recognition, speech recognition, and natural language generation.
AI has been integrated into various aspects of the financial sector, such as:
- Banking: AI is helping banks to enhance their customer service, streamline their operations, reduce their costs, and increase their revenues. Some of the applications of AI in banking are:
- Chatbots: These are conversational agents that can interact with customers via text or voice, and provide information, guidance, and assistance. Chatbots can help banks to improve their customer satisfaction, retention, and loyalty, as well as to reduce their operational expenses and human errors.
- Robo-advisors: These are automated platforms that can provide financial advice and investment management to customers, based on their goals, preferences, and risk profiles. Robo-advisors can help banks to expand their customer base, diversify their product offerings, and lower their fees and commissions.
- Fraud detection: This is the process of identifying and preventing fraudulent transactions and activities, such as identity theft, money laundering, and cyberattacks. Fraud detection can help banks to protect their assets, reputation, and customers, as well as to comply with the regulatory requirements and standards.
- Insurance: AI is enabling insurance companies to improve their underwriting, pricing, claims processing, and customer service. Some of the applications of AI in insurance are:
- Risk assessment: This is the process of evaluating the likelihood and severity of potential losses and damages, based on the characteristics and behavior of the insured entities. Risk assessment can help insurance companies to optimize their premiums, policies, and reserves, as well as to prevent adverse selection and moral hazard.
- Claims management: This is the process of handling and settling the claims made by the insured entities, in case of an event or occurrence that triggers the insurance coverage. Claims management can help insurance companies to speed up their claims processing, reduce their operational costs, and enhance their customer satisfaction.
- Personalization: This is the process of tailoring the insurance products and services to the specific needs and preferences of the customers. Personalization can help insurance companies to increase their customer loyalty, retention, and acquisition, as well as to differentiate themselves from the competitors.
- Wealth management: AI is empowering wealth managers and investors to make better and smarter financial decisions, based on data-driven insights and recommendations. Some of the applications of AI in wealth management are:
- Portfolio optimization: This is the process of selecting and allocating the optimal mix of assets and securities, based on the objectives, constraints, and risk preferences of the investors. Portfolio optimization can help wealth managers and investors to maximize their returns, minimize their risks, and diversify their investments.
- Market analysis: This is the process of examining and interpreting the trends, patterns, and movements of the financial markets, based on the historical and current data and information. Market analysis can help wealth managers and investors to identify and exploit the opportunities, anticipate and avoid the threats, and adjust and adapt their strategies.
- Sentiment analysis: This is the process of extracting and measuring the opinions, emotions, and attitudes of the market participants, based on the textual and vocal data and information. Sentiment analysis can help wealth managers and investors to gauge the market sentiment, predict the market behavior, and enhance their decision-making.
Predictive Analytics in Finance
Predictive analytics is one of the most prominent and powerful applications of AI in finance. Predictive analytics is the process of using data, statistical models, and machine learning techniques to forecast future outcomes and trends. Predictive analytics can help financial institutions and investors to gain a competitive edge, improve their performance, and reduce their risks.
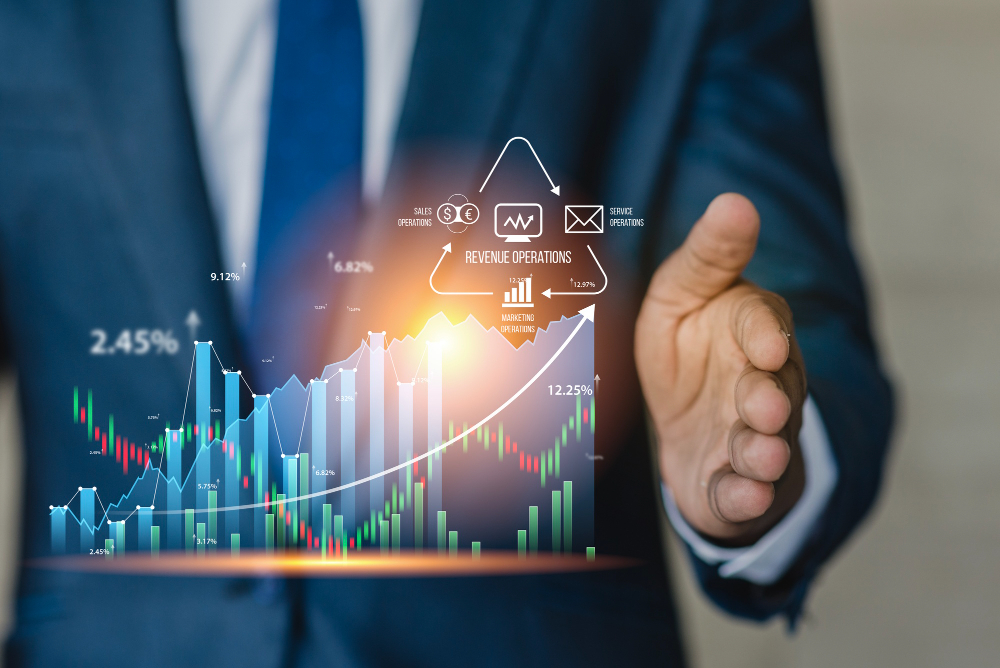
Predictive analytics can be applied in various aspects of financial decision-making, such as:
- Risk assessment and management: This is the process of identifying, measuring, and mitigating the potential losses and damages that may arise from the uncertainty and volatility of the financial markets and activities. Risk assessment and management can help financial institutions and investors to protect their capital, reputation, and customers, as well as to comply with the regulatory requirements and standards. Some of the examples of risk assessment and management using predictive analytics are:
- Credit scoring: This is the process of evaluating the creditworthiness and default probability of the borrowers, based on their financial history, behavior, and characteristics. Credit scoring can help lenders to approve or reject the loan applications, determine the interest rates and terms, and monitor the repayment performance.
- Stress testing: This is the process of simulating the impact of various adverse scenarios and events on the financial position and performance of the financial institutions and investors. Stress testing can help them to assess their resilience, solvency, and liquidity, as well as to prepare and implement their contingency plans.
- Value at risk (VaR): This is a measure of the maximum potential loss that a portfolio or a position may incur over a given period of time, with a given level of confidence. VaR can help financial institutions and investors to quantify their exposure, allocate their capital, and limit their losses.
- Customer behavior analysis and segmentation: This is the process of understanding and predicting the preferences, needs, and actions of the customers, based on their demographic, psychographic, and behavioral data and information. Customer behavior analysis and segmentation can help financial institutions to enhance their customer service, retention, and loyalty, as well as to increase their revenues and profits. Some of the examples of customer behavior analysis and segmentation using predictive analytics are:
- Churn prediction: This is the process of identifying and preventing the customers who are likely to stop using the products or services of the financial institutions. Churn prediction can help them to reduce their customer attrition, increase their customer lifetime value, and improve their customer satisfaction.
- Cross-selling and up-selling: These are the processes of offering and selling additional or complementary products or services to the existing or potential customers, based on their purchase history, behavior, and preferences. Cross-selling and up-selling can help financial institutions to increase their customer base, diversify their product offerings, and boost their revenues and profits.
- Customer segmentation: This is the process of dividing the customers into homogeneous groups or segments, based on their similarities and differences in terms of their characteristics, behavior, and needs. Customer segmentation can help financial institutions to tailor their products and services, marketing and communication, and pricing and promotion strategies, to the specific needs and preferences of each segment.
Algorithmic Trading Strategies
Another important and influential application of AI in finance is in algorithmic trading. Algorithmic trading is the use of computer programs and algorithms to execute trading orders based on predefined rules and strategies. Algorithmic trading can help financial institutions and investors to enhance their trading performance, reduce their trading costs, and increase their trading speed and efficiency.
Algorithmic trading can be classified into different types, based on the logic, frequency, and duration of the trading strategies. Some of the common types of algorithmic trading strategies are:
- Trend-following strategies: These are strategies that aim to exploit the persistent and consistent movements of the market prices in a certain direction, either upward or downward. Trend-following strategies can use various indicators and signals, such as moving averages, trend lines, and breakouts, to identify and follow the market trends. Trend-following strategies can help traders to capture the large and long-term profits from the market movements, as well as to avoid the market noise and fluctuations.
- Mean reversion strategies: These are strategies that assume that the market prices tend to revert to their mean or average values over time, after deviating from them due to various factors, such as overreaction, underreaction, or random events. Mean reversion strategies can use various tools and techniques, such as Bollinger bands, oscillators, and arbitrage, to measure and exploit the deviations of the market prices from their mean values. Mean reversion strategies can help traders to profit from the short-term and small-scale corrections and reversals of the market prices, as well as to diversify their portfolio and reduce their risk.
- High-frequency trading (HFT) algorithms: These are algorithms that use advanced technology and infrastructure, such as high-speed computers, networks, and data feeds, to execute a large number of trading orders within a very short period of time, ranging from milliseconds to seconds. HFT algorithms can use various methods and tactics, such as market making, liquidity provision, order anticipation, and statistical arbitrage, to exploit the market inefficiencies, opportunities, and anomalies. HFT algorithms can help traders to generate high returns, increase market liquidity, and improve market efficiency.
Algorithmic trading has a significant impact on the financial markets, as it accounts for a large and growing proportion of the trading volume and activity. According to some estimates, algorithmic trading represents more than 70% of the trading volume in the US equity markets, and more than 50% of the trading volume in the global forex markets . Algorithmic trading can affect the market dynamics, behavior, and structure, as it can influence the market prices, volatility, liquidity, and competition.
AI-Powered Predictive Analytics Tools
The increasing demand and adoption of AI-powered predictive analytics in finance has led to the development and emergence of various platforms and tools that can provide data-driven insights and recommendations to the financial institutions and investors. These platforms and tools can leverage the power of AI technologies, such as machine learning, deep learning, natural language processing, and computer vision, to analyze and process large and complex data sets, and generate accurate and reliable predictions and forecasts.
Some of the features and capabilities of the leading AI-driven predictive analytics platforms and tools are:
- Data integration and ingestion: These are the processes of collecting, connecting, and importing data from various sources and formats, such as databases, files, APIs, and web pages, into a unified and standardized data platform or tool. Data integration and ingestion can help to ensure the quality, consistency, and completeness of the data, as well as to facilitate the data access and analysis.
- Data exploration and visualization: These are the processes of examining, understanding, and presenting the data, using various techniques and tools, such as charts, graphs, tables, and dashboards. Data exploration and visualization can help to discover and highlight the patterns, trends, and outliers in the data, as well as to communicate and share the data insights and findings.
- Data modeling and analysis: These are the processes of applying various statistical models and machine learning algorithms to the data, in order to perform various tasks and operations, such as classification, regression, clustering, and anomaly detection. Data modeling and analysis can help to extract and derive the meaningful and actionable information and knowledge from the data, as well as to evaluate and validate the data results and outcomes.
- Data prediction and forecasting: These are the processes of using the data models and analysis to estimate and project the future values and scenarios of the data variables and indicators, based on the historical and current data and information. Data prediction and forecasting can help to anticipate and prepare for the future events and occurrences, as well as to optimize and improve the decision-making and planning.
- Data reporting and recommendation: These are the processes of generating and delivering the data reports and recommendations, based on the data exploration, visualization, modeling, analysis, prediction, and forecasting. Data reporting and recommendation can help to provide the data insights and suggestions, as well as to support and guide the data-driven actions and strategies.
Some of the case studies showcasing the successful implementation of AI in predictive analytics within finance are:
- American Express: American Express is a global financial services company that offers various products and services, such as credit cards, charge cards, travel, and insurance. American Express uses AI-powered predictive analytics to enhance its customer service, retention, and loyalty, as well as to increase its revenues and profits. Some of the applications of AI in predictive analytics by American Express are:
- Customer churn prediction: American Express uses machine learning models to analyze the customer data and behavior, and identify the customers who are likely to cancel their cards or switch to other providers. American Express then uses targeted marketing and communication campaigns, as well as personalized offers and incentives, to retain and engage the customers, and prevent them from churning.
- Customer lifetime value prediction: American Express uses machine learning models to estimate the future value and profitability of each customer, based on their purchase history, behavior, and preferences. American Express then uses this information to segment and prioritize the customers, as well as to tailor and optimize its products and services, pricing and promotion strategies, and cross-selling and up-selling opportunities, to each customer segment.
- JP Morgan Chase: JP Morgan Chase is a multinational banking and financial services company that offers various products and services, such as investment banking, asset management, commercial banking, and consumer banking. JP Morgan Chase uses AI-powered predictive analytics to improve its risk assessment and management, as well as to reduce its costs and errors. Some of the applications of AI in predictive analytics by JP Morgan Chase are:
- Credit risk prediction: JP Morgan Chase uses machine learning models to evaluate the credit risk and default probability of the borrowers, based on their financial history, behavior, and characteristics. JP Morgan Chase then uses this information to approve or reject the loan applications, determine the interest rates and terms, and monitor the repayment performance.
- Fraud detection: JP Morgan Chase uses machine learning models to detect and prevent fraudulent transactions and activities, such as identity theft, money laundering, and cyberattacks. JP Morgan Chase then uses this information to protect its assets, reputation, and customers, as well as to comply with the regulatory requirements and standards.