Home to training index page > AI trainings > Data Science
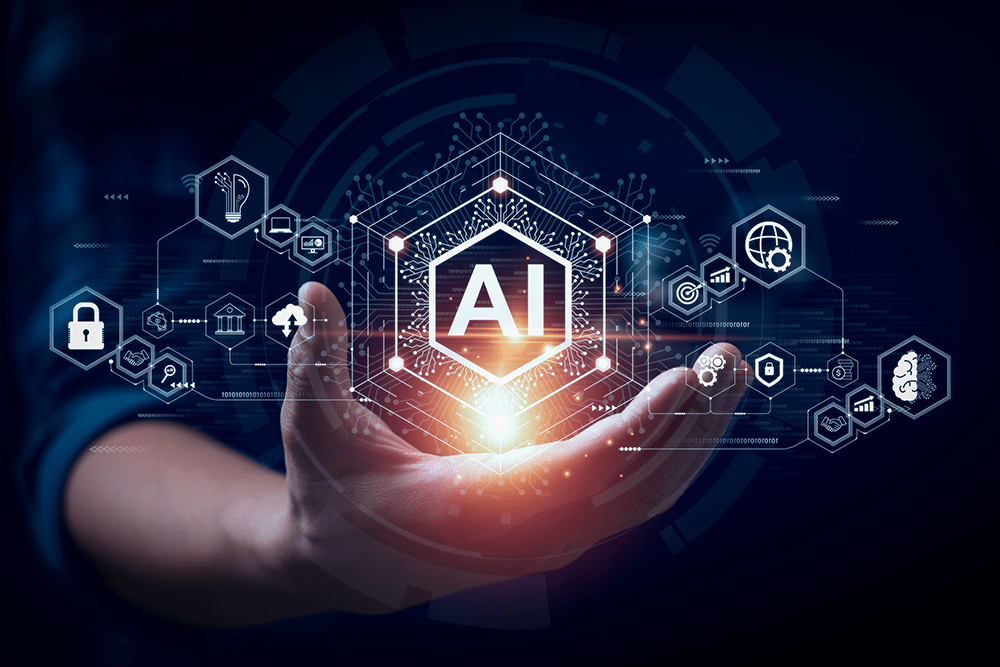
In the ever-evolving landscape of technology, the marriage of Artificial Intelligence (AI) and Data Science has emerged as a powerful union, reshaping the way we perceive and harness information. Data Science, a field that revolves around statistical and computational methodologies to dissect vast datasets, has become intrinsically linked with AI components. This symbiotic relationship not only extends the capabilities of both disciplines but also fuels advancements in machine learning, deep learning, data visualization, mining, and predictive modeling. In this comprehensive exploration, we delve into the intricate connections between AI and Data Science, unraveling the transformative impact they wield across industries and society.
I. The Foundation of Data Science
- Defining Data Science:
At its core, Data Science is the art of extracting meaningful insights from complex and large datasets. It combines statistical and computational methods to uncover patterns, trends, and correlations that drive informed decision-making. From business analytics to scientific research, Data Science has permeated various domains, providing a systematic approach to understanding and interpreting data. - Key Components of Data Science:
Data Science encompasses a diverse range of techniques and methodologies, including data visualization, data mining, and predictive modeling. Visualization aids in presenting data in a comprehensible format, while data mining involves extracting valuable patterns from datasets. Predictive modeling utilizes statistical algorithms to forecast future trends based on historical data, offering valuable insights for strategic planning.
II. The Integration of AI into Data Science
- Machine Learning and Data Science:
One of the pivotal components of AI integrated into Data Science is machine learning. It enables systems to learn and improve from experience without explicit programming. Supervised learning, unsupervised learning, and reinforcement learning are prominent branches of machine learning, each offering unique capabilities in data analysis, pattern recognition, and decision-making. - Deep Learning’s Ascendance:
Deep Learning, a subset of machine learning, has garnered immense attention for its prowess in handling unstructured data, such as images, audio, and text. Neural networks, inspired by the human brain’s architecture, form the foundation of deep learning models. This revolutionary approach has fueled breakthroughs in image recognition, natural language processing, and autonomous systems.
III. The Nexus of AI and Data Science in Practice
- Data Science in AI Development:
Data Science plays a pivotal role in developing and optimizing AI algorithms. By feeding machine learning models with vast datasets, Data Scientists contribute to the training and improvement of AI systems. This iterative process refines the algorithms, enhancing their accuracy, efficiency, and adaptability to diverse scenarios. - AI’s Impact on Data Science Processes:
The integration of AI has transformed traditional Data Science processes. Automated machine learning (AutoML) has streamlined model selection, hyperparameter tuning, and feature engineering, reducing the manual effort required. This evolution accelerates the pace of insights generation, enabling Data Scientists to focus on higher-level tasks and strategic decision-making.
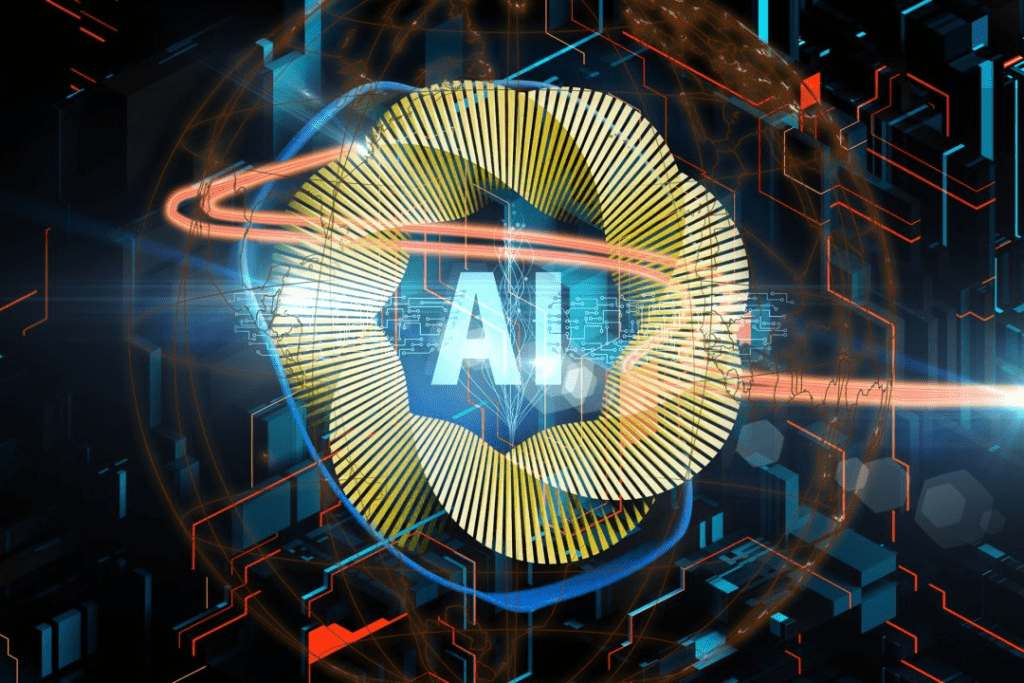
IV. Data Science Training: An Inclusive Approach to AI
- Comprehensive Curricula:
Many contemporary Data Science training programs have embraced AI as an integral component of their curricula. These programs go beyond the rudimentary statistical methodologies, incorporating in-depth studies of machine learning, deep learning, and AI frameworks. This holistic approach equips aspiring Data Scientists with the skills needed to navigate the intricacies of AI applications. - Hands-On Experience:
Effective Data Science training involves hands-on experience with real-world datasets and AI tools. Practical applications in machine learning, where students build and deploy models, foster a deeper understanding of the synergy between Data Science and AI. This experiential learning approach ensures graduates are well-prepared for the dynamic landscape of data-driven industries.
V. AI and Data Science Metrics: A Comparative Analysis
- Growth Metrics:
Evaluating the growth trajectories of AI and Data Science involves analyzing key metrics such as job demand, market investments, and technological advancements. The demand for skilled professionals in both fields has skyrocketed, reflecting the pivotal role they play in shaping industries. Concurrently, substantial investments in research and development underscore the commitment to fostering innovation. - Technological Advancements:
The technological advancements in AI and Data Science are intertwined, each catalyzing the progress of the other. From the refinement of algorithms to the emergence of sophisticated tools and frameworks, the collaborative evolution of AI and Data Science continues to push the boundaries of what is achievable in data analysis, pattern recognition, and decision-making.
VI. AI and Data Science Applications Across Industries
- Healthcare:
In healthcare, the integration of AI and Data Science has revolutionized diagnostics, treatment plans, and patient care. Predictive modeling aids in identifying potential outbreaks, while machine learning algorithms analyze medical imaging for early disease detection. These applications enhance the efficiency and accuracy of healthcare systems. - Finance:
The finance sector leverages AI and Data Science for fraud detection, risk assessment, and algorithmic trading. Predictive analytics guides investment strategies, while machine learning models analyze transaction patterns to identify anomalies. The synergy of AI and Data Science has ushered in a new era of data-driven decision-making in the financial landscape. - Retail and E-Commerce:
In the retail and e-commerce sectors, AI and Data Science optimize inventory management, enhance customer experiences, and personalize recommendations. Predictive analytics anticipates consumer trends, while machine learning algorithms analyze purchasing behaviors, refining marketing strategies and increasing customer engagement. - Manufacturing and Supply Chain:
AI and Data Science applications in manufacturing and supply chain operations include predictive maintenance, quality control, and demand forecasting. Data-driven insights facilitate efficient resource allocation, reduce downtime, and enhance overall operational efficiency.
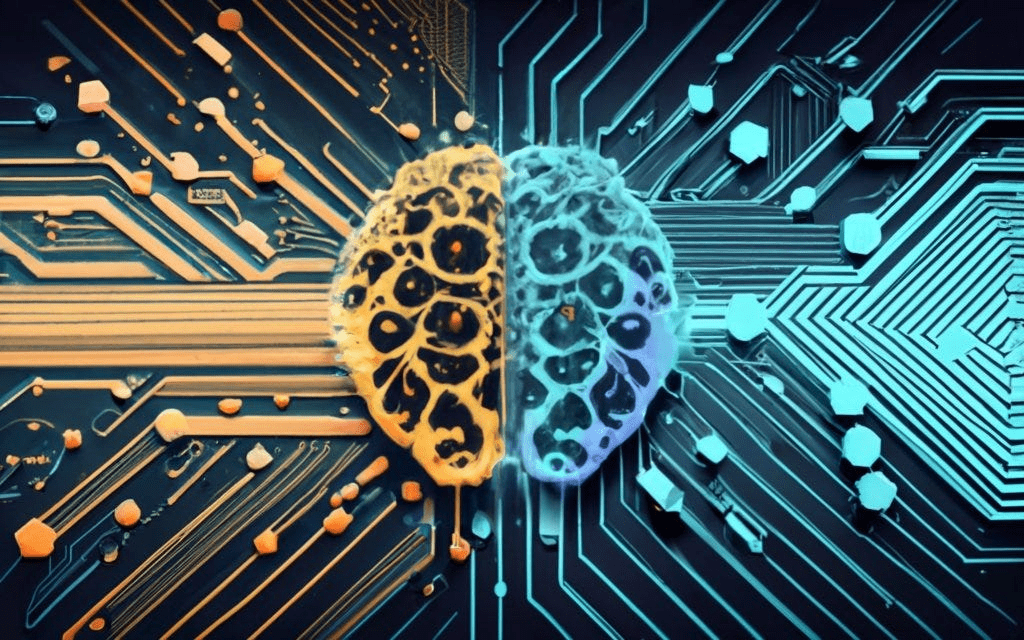
VII. Ethical Considerations and Challenges
- Data Privacy and Bias:
The integration of AI into Data Science brings forth ethical considerations, particularly concerning data privacy and bias. Ensuring responsible and transparent use of data is paramount to mitigating privacy concerns, while addressing biases in algorithms requires ongoing vigilance to prevent inadvertent discrimination. - Interpretable AI Models:
The black-box nature of some AI models poses challenges in terms of interpretability. Striking a balance between model complexity and interpretability is crucial for ensuring trust and understanding, especially in fields where decisions impact human lives, such as healthcare and criminal justice.
VIII. Future Perspectives and Innovations
- Explainable AI:
The push for more transparent AI models has led to the development of Explainable AI. This emerging field aims to create models that not only make accurate predictions but also provide understandable justifications for their decisions. This enhances trust, accountability, and ethical considerations in AI applications. - AI-Enhanced Data Science Tools:
The future of Data Science will likely see the integration of AI-enhanced tools that automate routine tasks, accelerate insights generation, and offer more sophisticated analytics capabilities. This shift allows Data Scientists to focus on strategic decision-making, innovation, and addressing complex challenges.
In Short
As we navigate the intricate interplay between Artificial Intelligence and Data Science, it becomes evident that their synergy is reshaping industries, fostering innovation, and driving the evolution of technology. The comprehensive exploration presented in this article underscores the inseparable bond between AI and Data Science, from their foundational principles to their far-reaching applications across diverse sectors. As we step into the future, the collaborative advancements in these fields hold
Related Content
- 10 Best Free Machine Learning Courses for Beginners to Join in 2023
- Great Learning – Free Machine Learning courses
- JIRA basis trainings
- Trello basis trainings and project management
- Project Management tools trainings
- The Synergy of Artificial Intelligence and Data Science: Unveiling the Future of Innovation
- Data Science and Artificial Intelligence: A Powerful Duo | LinkedIn
- Unveiling the Synergy: Exploring Advances in Applied Artificial Intelligence and Narrow AI
- Unveiling the Synergy of Artificial Intelligence and Data Science: A Comprehensive Exploration
If you need assistance with real-life scenarios or recommendations, please feel free to contact us either HERE or through email at trainings@micro2media.com.