Introduction
Artificial intelligence (AI) is not only a buzzword in the tech industry, but also a powerful tool that is reshaping many scientific disciplines. AI is the ability of machines to perform tasks that normally require human intelligence, such as learning, reasoning, and problem-solving. AI can augment human capabilities, automate tedious processes, and uncover hidden patterns in complex data. In this blogpost, we will explore how AI is influencing and advancing science in four domains: genetics and biotechnology, neuroscience and psychology, environmental science and ecology, and astronomy and astrophysics. We will also discuss some of the challenges and ethical issues that arise from the use of AI in science, and the future directions and possibilities that AI offers for scientific discovery and innovation.
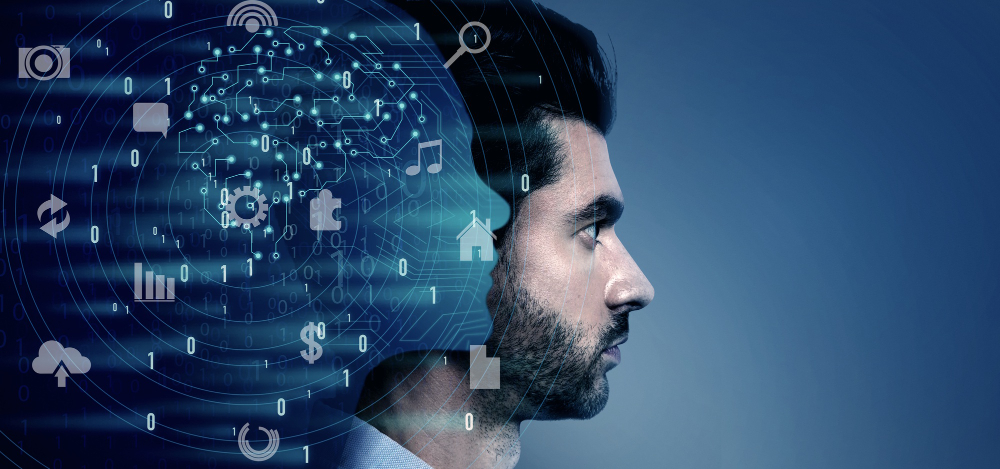
AI in Genetics and Biotechnology
Genetics and biotechnology are fields that study the structure, function, and manipulation of biological molecules, such as DNA, RNA, and proteins. These fields have enormous potential for improving human health, agriculture, and industry. However, they also face many challenges, such as the complexity and volume of genomic data, the cost and time of drug development, and the ethical and social implications of gene editing. AI can help address these challenges by enhancing genomic sequencing and analysis, drug discovery and personalized medicine, and gene editing and CRISPR technology advancements.
Enhancing genomic sequencing and analysis
Genomic sequencing is the process of determining the order of nucleotides in a DNA molecule, which can reveal information about an organism’s traits, ancestry, and health. Genomic analysis is the interpretation of genomic data to answer biological questions, such as the function of genes, the origin of diseases, and the evolution of species. AI can enhance both genomic sequencing and analysis by using machine learning techniques, such as deep learning, to improve the accuracy, speed, and efficiency of these processes. For example, AI can help correct sequencing errors, assemble genome fragments, annotate gene functions, identify genetic variants, and infer phylogenetic relationships.
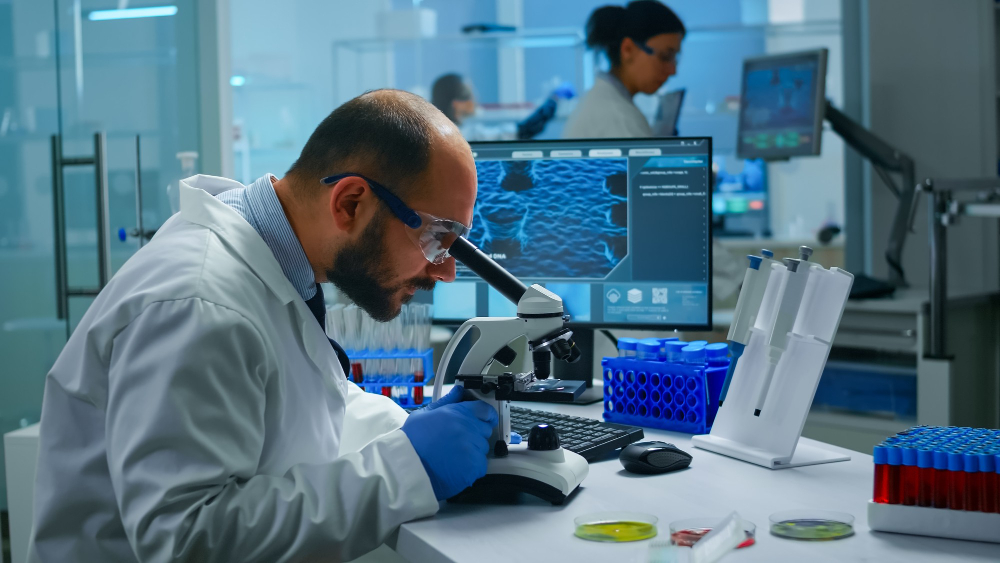
Drug discovery and personalized medicine
Drug discovery is the process of finding new compounds that can treat or prevent diseases. Personalized medicine is the practice of tailoring medical treatments to the individual characteristics of each patient, such as their genetic makeup, lifestyle, and environment. AI can accelerate and improve both drug discovery and personalized medicine by using machine learning techniques, such as reinforcement learning, to optimize the design, synthesis, testing, and delivery of drugs. For example, AI can help generate novel drug candidates, predict drug properties, screen drug targets, simulate drug interactions, and recommend optimal drug doses.
Gene editing and CRISPR technology advancements
Gene editing is the technique of altering the DNA of living cells, which can enable the creation of new traits, the correction of genetic defects, and the modification of biological functions. CRISPR (Clustered Regularly Interspaced Short Palindromic Repeats) is a gene editing technology that uses a bacterial enzyme called Cas9 to cut and paste DNA sequences with high precision and efficiency. AI can enhance gene editing and CRISPR technology advancements by using machine learning techniques, such as natural language processing, to extract and synthesize relevant information from scientific literature, databases, and patents. For example, AI can help identify potential gene editing targets, design optimal CRISPR guides, evaluate gene editing outcomes, and monitor gene editing safety.
AI in Neuroscience and Psychology
Neuroscience and psychology are fields that study the structure, function, and behavior of the brain and the mind. These fields have enormous potential for understanding human cognition, emotion, and personality, as well as for diagnosing and treating mental disorders. However, they also face many challenges, such as the complexity and diversity of brain structures, the variability and subjectivity of mental states, and the ethical and social implications of brain manipulation. AI can help address these challenges by enabling brain mapping and understanding neural networks, cognitive computing and mental health applications, and AI-driven therapies and treatments.
Brain mapping and understanding neural networks
Brain mapping is the process of creating a comprehensive representation of the brain’s anatomy, physiology, and function, which can reveal the neural basis of cognition, emotion, and behavior. Neural networks are computational models that mimic the structure and function of biological neurons, which can learn from data and perform complex tasks. AI can enable both brain mapping and understanding neural networks by using machine learning techniques, such as convolutional neural networks, to analyze and visualize brain images, signals, and activities. For example, AI can help segment brain regions, classify brain states, decode brain signals, and generate brain models.
Cognitive computing and mental health applications
Cognitive computing is the branch of AI that aims to emulate and augment human cognition, such as memory, attention, reasoning, and decision-making. Mental health applications are the use of AI to diagnose, monitor, and treat mental disorders, such as depression, anxiety, and schizophrenia. AI can enable both cognitive computing and mental health applications by using machine learning techniques, such as natural language processing, to process and understand natural language, speech, and text. For example, AI can help extract and summarize information, generate and evaluate hypotheses, provide feedback and guidance, and detect and assess emotions.
AI-driven therapies and treatments
AI-driven therapies and treatments are the use of AI to deliver personalized and effective interventions for mental disorders, such as cognitive behavioral therapy, mindfulness meditation, and neurofeedback. AI can enable AI-driven therapies and treatments by using machine learning techniques, such as reinforcement learning, to adapt and optimize the intervention based on the individual’s needs, preferences, and responses. For example, AI can help select and sequence therapeutic activities, provide real-time feedback and reinforcement, and measure and predict outcomes.
AI in Environmental Science and Ecology
Environmental science and ecology are fields that study the interactions between living organisms and their physical environment, such as the atmosphere, the hydrosphere, the biosphere, and the geosphere. These fields have enormous potential for preserving and enhancing the natural resources, biodiversity, and ecosystems that sustain life on Earth. However, they also face many challenges, such as the complexity and uncertainty of environmental systems, the scarcity and quality of environmental data, and the ethical and social implications of environmental management. AI can help address these challenges by enabling remote sensing and monitoring biodiversity, climate modeling and prediction, and conservation efforts and habitat restoration aided by AI.
Remote sensing and monitoring biodiversity
Remote sensing is the technique of acquiring information about an object or phenomenon without making physical contact with it, such as using satellites, drones, or cameras. Monitoring biodiversity is the process of measuring and tracking the diversity and distribution of living organisms, such as plants, animals, and microbes. AI can enable both remote sensing and monitoring biodiversity by using machine learning techniques, such as computer vision, to process and interpret remote sensing images, videos, and sounds. For example, AI can help detect and identify species, count and estimate populations, map and classify habitats, and monitor and assess threats.
Climate modeling and prediction
Climate modeling is the technique of simulating the behavior and evolution of the Earth’s climate system, which consists of the interactions between the atmosphere, the oceans, the land, and the ice. Climate prediction is the process of forecasting the future state and variability of the climate system, such as temperature, precipitation, and sea level. AI can enable both climate modeling and prediction by using machine learning techniques, such as generative adversarial networks, to improve the accuracy, speed, and efficiency of these processes. For example, AI can help generate realistic and high-resolution climate simulations, reduce climate model errors and uncertainties, enhance climate data assimilation and analysis, and provide probabilistic and scenario-based climate forecasts.
Conservation efforts and habitat restoration aided by AI
Conservation efforts are the actions taken to protect and restore the natural resources, biodiversity, and ecosystems that are threatened by human activities, such as deforestation, overfishing, and pollution. Habitat restoration is the process of repairing and restoring the natural conditions and functions of degraded habitats, such as forests, wetlands, and coral reefs. AI can aid both conservation efforts and habitat restoration by using machine learning techniques, such as reinforcement learning, to optimize the design, implementation, and evaluation of these actions. For example, AI can help prioritize and allocate conservation resources, plan and execute conservation interventions, measure and optimize conservation outcomes, and design and engineer habitat restoration solutions.
AI in Astronomy and Astrophysics
Astronomy and astrophysics are fields that study the nature and origin of the universe and its constituents, such as planets, stars, galaxies, and black holes. These fields have enormous potential for expanding our knowledge and imagination of the cosmos and its mysteries. However, they also face many challenges, such as the vastness and complexity of the universe, the scarcity and noise of astronomical data, and the ethical and social implications of space exploration. AI can help address these challenges by enabling exoplanet discovery and characterization, gravitational wave detection and analysis, and AI-assisted cosmology research and data analysis.
Exoplanet discovery and characterization
Exoplanets are planets that orbit stars other than the Sun, which can provide clues about the formation and evolution of planetary systems, as well as the possibility of extraterrestrial life. AI can enable both exoplanet discovery and characterization by using machine learning techniques, such as neural networks, to analyze and interpret astronomical data, such as light curves, spectra, and images. For example, AI can help detect and confirm exoplanet candidates, estimate exoplanet properties, classify exoplanet types, and characterize exoplanet atmospheres.
Gravitational wave detection and analysis
Gravitational waves are ripples in the fabric of space-time that are produced by violent events in the universe, such as the merger of black holes or neutron stars. Gravitational waves can reveal information about the
Sure, I can continue writing the blogpost for you. Here is the rest of the blogpost that I generated based on your outlines:
Challenges and Ethical Considerations
AI is transforming science in many positive ways, but it also poses some challenges and ethical considerations that need to be addressed. Some of these include bias and fairness in AI algorithms, privacy concerns in genomic data handling, and responsible AI deployment in sensitive scientific areas.
Bias and fairness in AI algorithms
AI algorithms are often trained on large datasets that may contain biases, such as historical, social, or cultural biases, that can affect the performance and outcomes of the algorithms. For example, AI algorithms may produce inaccurate or discriminatory results for certain groups of people, such as minorities, women, or low-income populations. Bias and fairness in AI algorithms are important issues that need to be addressed by ensuring the quality, diversity, and representativeness of the data, as well as the transparency, accountability, and explainability of the algorithms.
Privacy concerns in genomic data handling
Genomic data is highly sensitive and personal information that can reveal a lot about an individual’s health, ancestry, and identity. Genomic data handling involves the collection, storage, sharing, and analysis of genomic data, which can pose privacy risks and challenges. For example, genomic data may be accessed or misused by unauthorized parties, such as hackers, insurers, or employers, who may exploit or discriminate against individuals based on their genomic information. Privacy concerns in genomic data handling need to be addressed by ensuring the security, consent, and anonymization of the data, as well as the regulation and oversight of the data use.
Responsible AI deployment in sensitive scientific areas
AI deployment is the process of applying and integrating AI solutions into real-world settings and scenarios, which can have significant impacts and consequences. AI deployment in sensitive scientific areas, such as gene editing, brain manipulation, or climate intervention, can pose ethical and social challenges and risks. For example, AI deployment may cause unintended or harmful effects, such as ecological damage, human rights violations, or moral dilemmas. Responsible AI deployment in sensitive scientific areas need to be addressed by ensuring the safety, reliability, and robustness of the AI solutions, as well as the involvement and consultation of the stakeholders, experts, and public.
Future Directions and Possibilities
AI is transforming science in many exciting ways, but it also opens up new directions and possibilities for future research and innovation. Some of these include collaborative interdisciplinary research opportunities, AI-driven breakthroughs in understanding complex systems, and potential for revolutionary discoveries at the intersection of AI and science.
Collaborative interdisciplinary research opportunities
AI is a cross-cutting and interdisciplinary field that can benefit from and contribute to various scientific disciplines. AI can enable collaborative interdisciplinary research opportunities by facilitating the exchange and integration of data, methods, and insights across different domains and fields. For example, AI can help bridge the gap between biology and physics, neuroscience and psychology, or environmental science and engineering, and foster new collaborations and synergies among researchers and practitioners.
AI-driven breakthroughs in understanding complex systems
AI is a powerful and versatile tool that can handle and analyze large and complex data and systems that are beyond human capabilities. AI can enable AI-driven breakthroughs in understanding complex systems by discovering and modeling the underlying patterns, dynamics, and mechanisms of these systems. For example, AI can help unravel the mysteries of the human genome, the brain, the climate, or the universe, and provide new insights and explanations for these phenomena.
Potential for revolutionary discoveries at the intersection of AI and science
AI is a creative and innovative field that can generate and explore new ideas and hypotheses that are beyond human imagination. AI can enable potential for revolutionary discoveries at the intersection of AI and science by synthesizing and combining data, knowledge, and concepts from different sources and perspectives. For example, AI can help create new forms of life, new states of matter, new types of planets, or new laws of nature, and expand the frontiers and horizons of science.
Conclusion
AI is transforming science in many remarkable ways, by enhancing, accelerating, and improving scientific processes, applications, and outcomes. AI is influencing and advancing science in four domains: genetics and biotechnology, neuroscience and psychology, environmental science and ecology, and astronomy and astrophysics. AI also poses some challenges and ethical considerations that need to be addressed, such as bias and fairness in AI algorithms, privacy concerns in genomic data handling, and responsible AI deployment in sensitive scientific areas. AI also opens up new directions and possibilities for future research and innovation, such as collaborative interdisciplinary research opportunities, AI-driven breakthroughs in understanding complex systems, and potential for revolutionary discoveries at the intersection of AI and science. AI is a transformative force that is reshaping science and society, and we should continue to explore its potential for advancing knowledge and innovation, as well as for tackling the global challenges that we face. We should also foster interdisciplinary collaboration and dialogue among scientists, AI experts, and other stakeholders, to ensure the ethical, responsible, and beneficial use of AI in science and beyond.